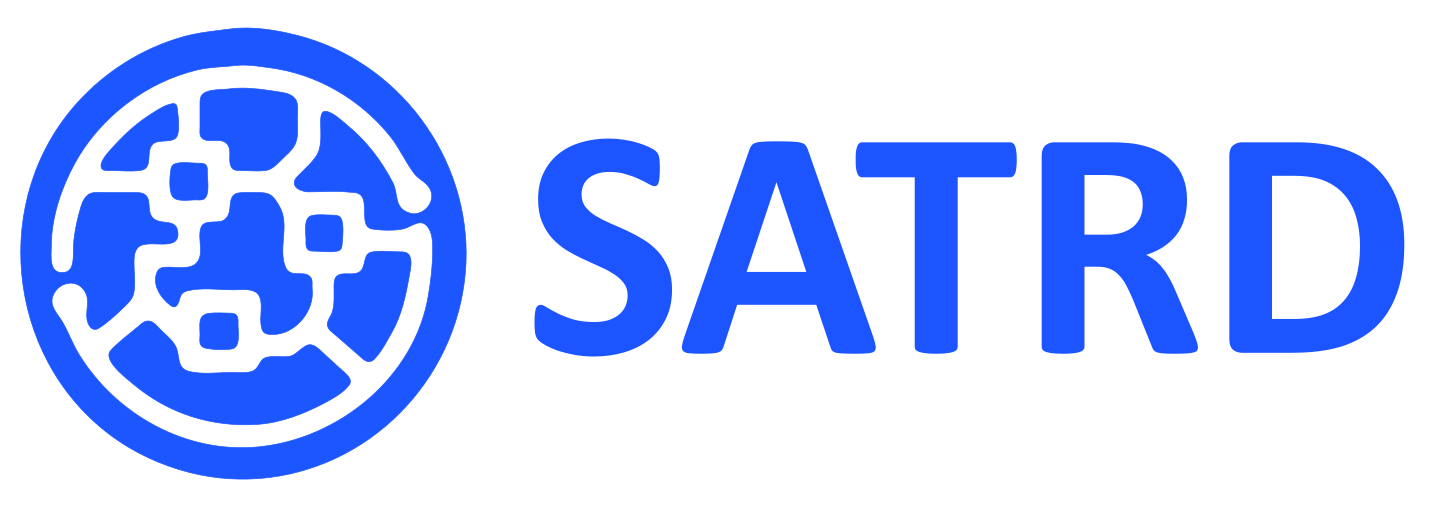
PROJECTS
ASIGNAT
The ASIGNAT project aims to design and develop tools that facilitate and enable better decision making in the operation of land transport of goods in port supply chains, establishing the following general objectives:
- Specify and develop a system for calculating and updating the ETA (Estimated Time of Arrival) in real time, with quality information obtained from transport information systems and on-board communications platforms, which will have the capacity to continuously send information from sensors to a central infrastructure in the cloud, with an open architecture.
- Design and develop intelligent tools based on machine learning for the optimal planning and allocation of multimodal routes, based on the collection and analysis of the history of all the information collected from the journeys. The incorporation of these tools will allow for decision support.
- Implement a digital twin model of the transport vehicle fleets that allows, being continuously connected to the physical objects, to update the data to reflect changes in the variables of the sensors of interest. This data will be used by machine learning algorithms for ETA prediction or assignment planning.
- Increase the degree of digitisation of existing processes in land transport companies, in turn obtaining optimum performance in transport operations, allowing the visualisation of information that helps decision-making. To this end, all the tools and algorithms will be integrated into a service architecture model that facilitates interconnection with the different data sources, as well as their sharing in a secure environment.
See Description in Spanish here
El proyecto ASIGNAT pretende diseñar y desarrollar herramientas que faciliten y habiliten una mejor toma de decisiones en las operaciones de transporte de mercancias terrestre en cadenas de suministro portuarias. Se han fijado los siguientes objetivos generales:
- Especificar y desarrollar un sistema para calcular y actualizar los valores de ETA (Estimated Time of Arrival) en tiempo real, con información de calidad obtenida por sistemas de información de transporte y plataformas de comunicación embarcadas, que tendrán la capacidad de enviar informacion de sensores a una infrastructura central en la nube, con una arquitectura abierta.
- Diseñar y desarrollar herramientas inteligentes basadas en machine learning para la planificación y fijación óptima de rutas multimodales, basado en la recolección y análisis del histórico de datos de las rutas.
- Implementar modelos de gemelo digital (digital twin) de las flotas de vehiculos de transporte, permitiendo: (i) conexión continua con los objetos fisicos, (ii) actualizacion de datos para reflejar los cambios en las variables de interés de los sensores. Estos datos serán usados por los algoritmos de machine learning para la predicción del valor de ETA o para planificar la asignación.
- Incrementar el grado de digitalización de los procesos existentes en las compañías de transporte terrestre y, de este modo, obtener el rendimiento óptimo en las operaciones de transporte, permitiendo la visualización de información que ayuda en la toma de decisión. Para este fin, todas las herramientas y algoritmos se integrarán en un modelo de arquitectura de servicios que facilita la interconexión con las distintas fuentes de datos, así como la compartición de estos datos en un entorno seguro.
El SATRD es responsable de la creación de una herramienta basada en un gemelo digital (Digital Twin) y Machine Learning para la optimización de flotas terrestres de transporte. Adicionalmente, se creará un mecanismo de recomendación para la asignación del tandem conductor-camión-trailer para cada orden de transporte basado en el estado en tiempo real de la flota, así como de reglas de negocio y optimización basado en inteligencia artificial (AI).
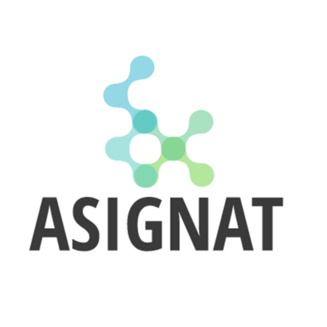

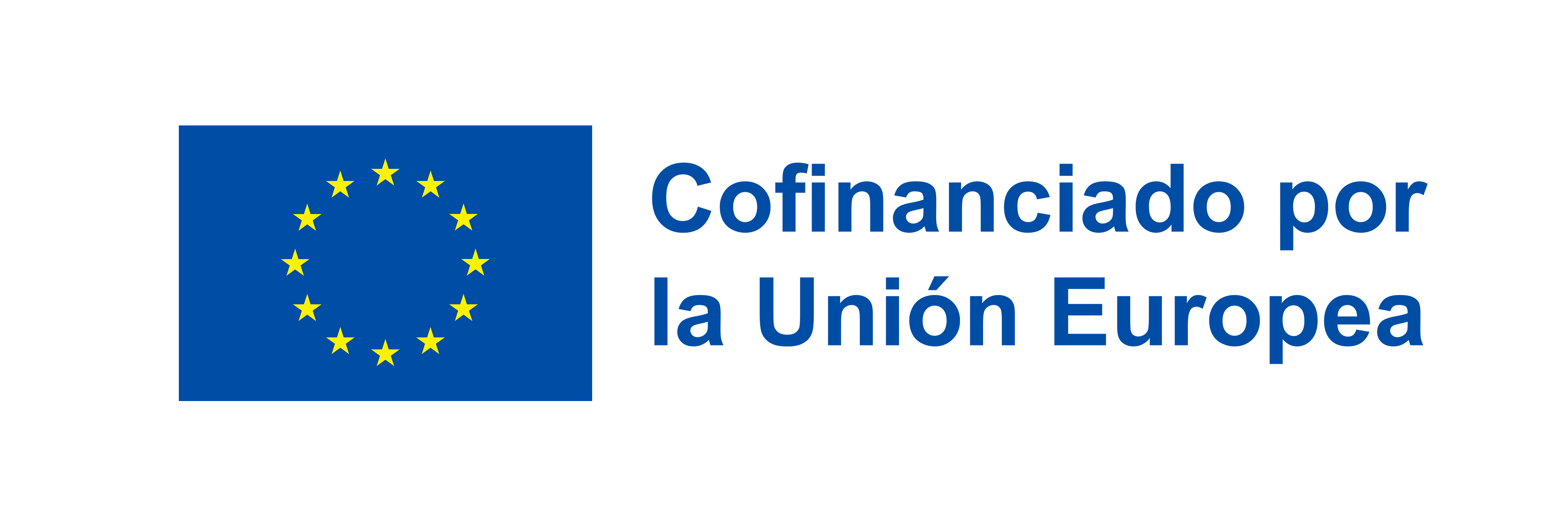
SATRD role in the ASIGNAT project
The SATRD is responsible for creating a tool based on Digital Twin and Machine Learning for the optimization of land transport fleets. In addition, it create a recommendation mechanism for the assignment of a driver-truck-trailer tandem for each transport order based on the real-time situation of the fleet and based on business rules and optimization based on AI.